What is the future of translation memory technology?
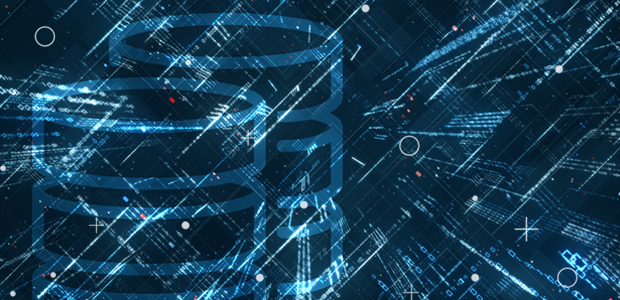
In part one of this blog, we touched on the history of translation memory (TM) and how Trados has approached things over the years in terms of development. In part two, we would like to take a more forward-thinking view of what some of the future developments in translation memory technology might bring.
For this, we sat down with two subject matter experts within Trados, Daniel Brockmann, Director of Product Management – a seasoned veteran in the translation industry, and Kevin Flanagan, Principal Research Engineer, with expertise in both software development and translation.
We asked Daniel and Kevin about their views on the future role TMs will play and to begin we picked probably the hottest topic of the moment: artificial intelligence (AI).
What role do you think AI could play in the future with translation memory?
DB: AI and machine learning is the topic everyone is talking about, from Siri and Alexa to self-driving cars to personal health tracking, AI is progressing rapidly. Trados already has some experience with machine learning – we launched our innovative self-learning adaptive machine translation technology back in 2016 – but the question arises about what it could mean for translation memory? Potentially we see the scenario where AI could deliver the next level in translation productivity increases – so at the very heart of what a CAT tool is.
One tangible aspect here could be improved translation suggestions. We could, for instance, imagine combining translation memory with terminology and machine translation (MT) results to always receive the best match to review, rather than translating from scratch. An example of this could be training a MT Translation Engine with a large TM and termbase from a certain subject matter area to increase the quality of MT even more for any specific use case. Another thought could be to augment an MT result with translation memory and terminology content, combining the best of both worlds.
In any event, the purpose would be to support, rather than replace, the human translator so that they can oversee the translation process more efficiently and fine-tune the initial results further.
KF: Beyond the core use case of translator productivity, AI could also improve project efficiency by performing an analysis of your TMs and recognizing which one of them is most relevant for your new project. For a project manager dealing with multiple projects and resources at the same time, this could be invaluable, again supporting them for more efficiently routing the content through the project supply chain.
How will translation memory work in the cloud in the future and what will be the benefits?
DB: The cloud-based way of working with TMs will provide exciting new possibilities for all participants in the supply chain. Finally, TM sharing will be democratized and possible for everyone to enjoy – from groups of freelancers working together via LSPs being able to share assets more easily, to large enterprises driving big translation projects. Those who will be able to integrate this way of working with a rich and powerful desktop environment will be the winners of this race. As an example, recent developments such as LookAhead in Trados Studio will provide a performance experience for cloud-based TMs that is the same, if not better, as working with local TMs located on the user’s hard disk.
How will TMs work within the augmented translation environment?
DB: Three main resources are now available for translators to make their job easier and more efficient. One – the traditional TM where nothing beats a 100% or context match. Two, the traditional terminology management to ensure quality and consistency of the translation at the term level. And now three, machine translation. With MT, for those language pairs where it is readily available, machine translation is now definitely ‘good enough’ to be fully and seamlessly plugged into the translation flow – not least since it is increasingly accepted by both work-givers and work-doers.
Now imagine these three resources working in tandem – a term could enhance an MT suggestion, or a MT fragment could augment a fuzzy match, etc. These are very exciting perspectives for leveraging years, if not decades, worth of building high-quality TMs and putting them in tandem with high-quality terminology and – this is where the shift is – high-quality machine translation. This does not mean that all translations will pop out perfectly from the machine. On the contrary – MT can be quite tricky in so far as it suggests a fluent translation. However, fluency does not necessarily mean accuracy. This is where the translator and reviewer come in and need to pay as much attention as possible to ensure that the translation will have the high quality that the customer will expect.
Having said all that, MT is an exciting new tool in the box for any translation flow for sure.
Translation memory vs machine translation – What is the future?
DB: It is likely there will be a new way of working with fuzzy matches. So what does this mean? An MT suggestion that may not need any editing at all could be better than a 70% or 80% fuzzy match that typically needs editing, even if it is a ‘repaired’ fuzzy match. So the future cadence could be: TM = best for 100% down to 90% fuzzy match, MT = best for 90% and lower. This, of course, will also have an impact on long-standing pricing models. Will work-givers look for a discount on new content as it is now reasonably well translated by an MT engine? Will work-doers readily accept this? Will there be a collaboration between both parties to reach a consensus?
Besides these budgetary questions, this also raises the question – are current editing environments optimized for working with TM combined with MT?
In the short term, what’s great is that CAT tool environments that are open platforms, such as Trados Studio, lend themselves perfectly to plugging in ‘any’ MT engine and continue working in well-known ways. So they are ideally suited for naturally adding MT to the mix of resources users have been working with anyway – and are familiar with – something that should never be underestimated. Also, current MT engines work at the segment level, which means that they naturally fit with the segment-based way of working.
In summary, at least in the short term, CAT tools like Trados Studio are a very good fit for simply ‘plugging in’ MT.
KF: With much higher quality coming in, in the longer term, we may need to rethink the grid-based editors. Changes could come with a different editing experience that is optimized for this new way of working, optimized for reviewing MT content rather than having lots of functionality for translation from scratch – which will no longer be needed in the same way as before. In the transition phase, however, we are already hearing from our customers that they simply add MT to the mix of resources in Trados Studio and optimize productivity and cost savings this way.
How can we make the user experience of working with translation memory better in the future?
KF: As well as re-thinking the editing experience, we see a future with a more document-centric approach to TM, so that for a TM match, you can drill down into the original document and translation, to see the entire document context.
What is the future for upLIFT?
KF: upLIFT translation memory is a feature that will continue improving. The quality of sub-segment suggestions, or ‘fragments’ as we call them, will get better with better word alignment and there will be enhanced handling of Match Repair cases. We will also be looking at:
- Alongside enhancing translation suggestions, upLIFT TM technology can provide a basis for better terminology extraction
- Automatic tag placement (even with match repair) developed from upLIFT building blocks
- Smarter TM maintenance based on upLIFT word alignment result that identifies mistranslations, data corruption or document/segment misalignments
As you can see, there are a lot of exciting potential developments on the horizon for translation memory technology, from AI to MT to the cloud.
What are you most excited about?